People
We, the people of the AIDOS Lab, firmly believe in and are committed to Dr. Federico Ardila-Mantilla’s axioms:
- Axiom 1. Mathematical potential is equally present in different groups, irrespective of geographic, demographic, and economic boundaries.
- Axiom 2. Everyone can have joyful, meaningful, and empowering mathematical experiences.
- Axiom 3. Mathematics is a powerful, malleable tool that can be shaped and used differently by various communities to serve their needs.
- Axiom 4. Every student deserves to be treated with dignity and respect.
All people are listed in chronological order of joining the lab or starting a collaboration with us.

Principal Investigator

Ph.D. Student

Ph.D. Student
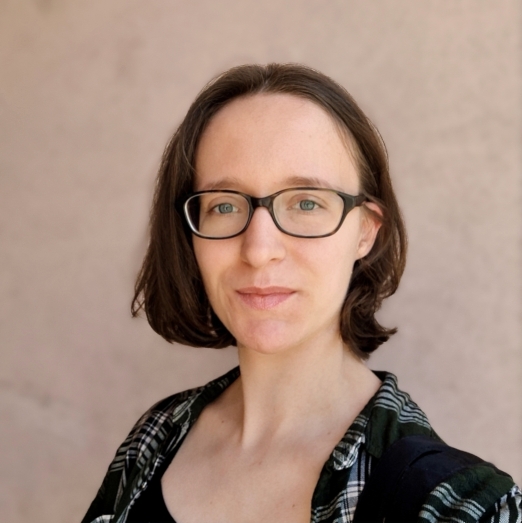
Ph.D. Student
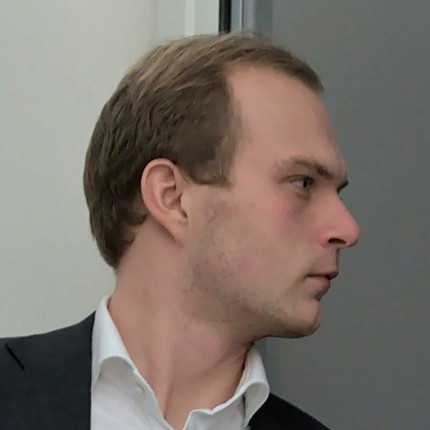
Ph.D. Student
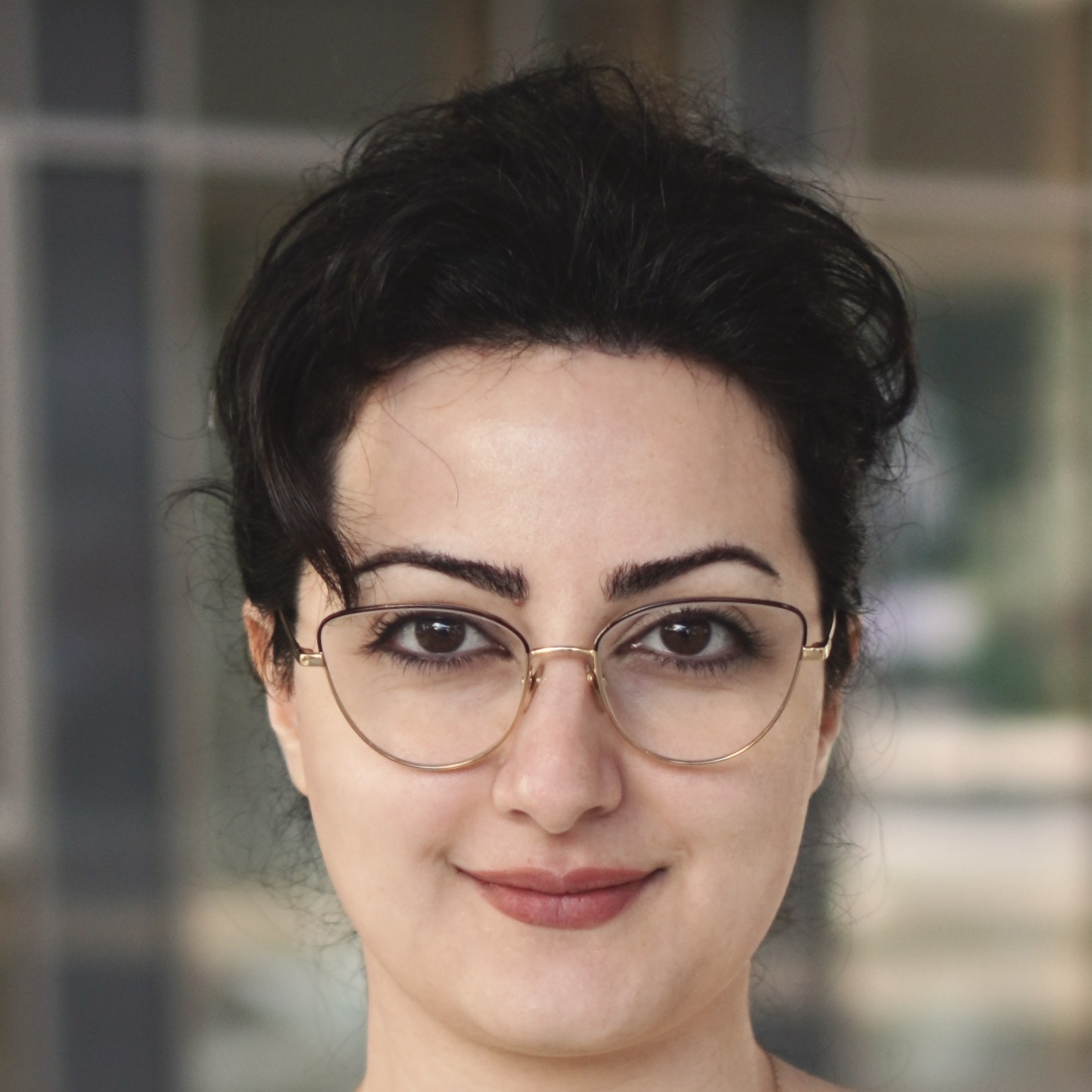
Ph.D. Student

Research Assistant

Ph.D. Student

Fulbright Student

Ph.D. Student

Ph.D. Student

Visiting Ph.D. Student

ELLIS Ph.D. Student

ELLIS Ph.D. Student
Collaborators
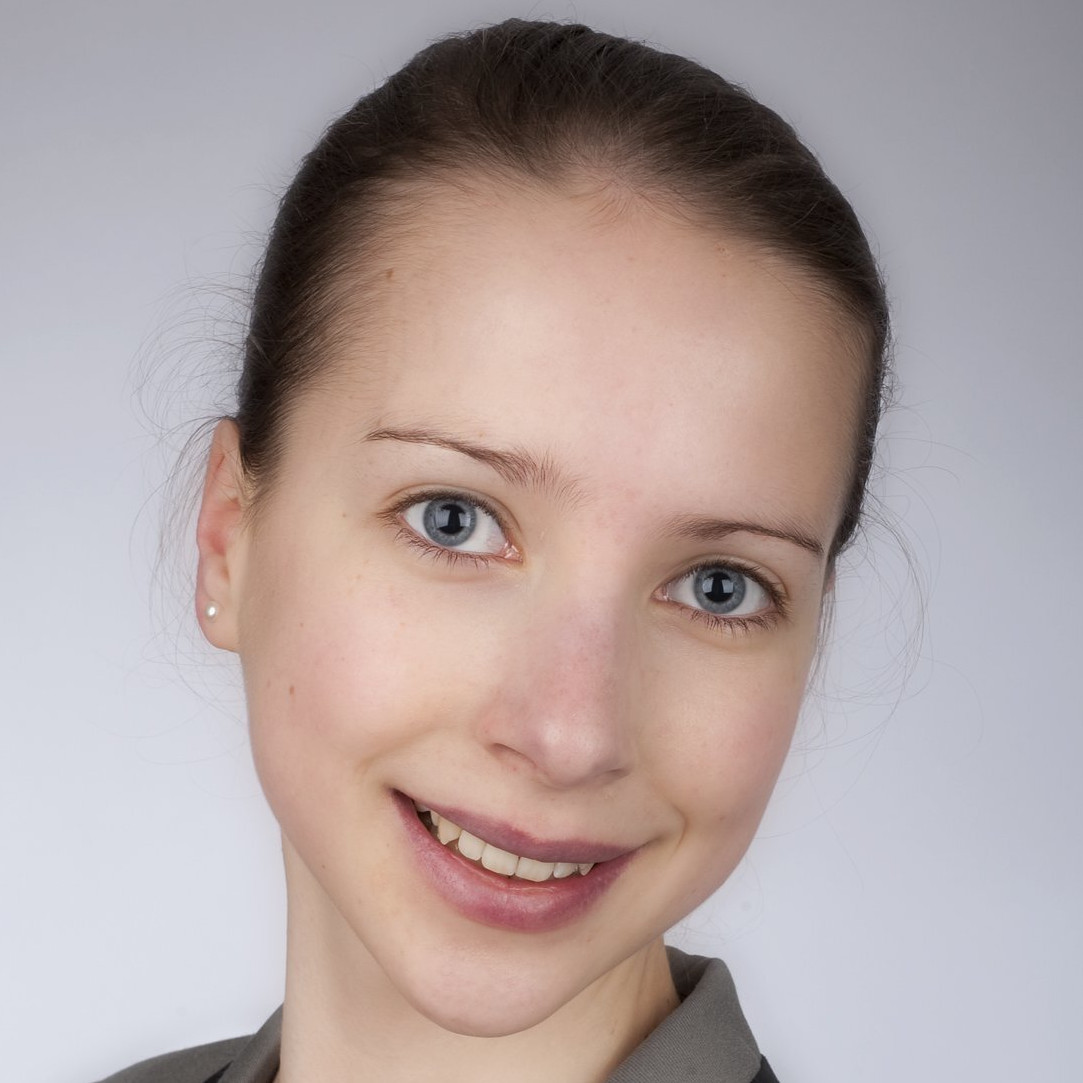


Your name is missing here! Learn more about joining us.
Alumni & Alumnae
- Pia Baronetzky
- Marek Cerny (now a Ph.D. student with Floris Geerts at the University of Antwerp)
- Ege Erdogan
- Katharina Hagedorn
- Ferdinand Hölzl (now a master’s student at University of Hamburg)
- Barış Onarıcı
- Yevhenii Sharapov
- Franz Srambical (now building AGI at p(doom))
- Tejas Srinivasan
- Kalyan Varma Nadimpalli (now a research assistant at IIT Madras)